Image Dehazing Transformer with Transmission-Aware 3D Position Embedding
2 Tianjin University, China
3 Australian National University, Australia
4 Beijing Jiaotong University, China
5 Sun Yat-sen University, China
6 Nanyang Technological University, Singapore
Abstract
Despite single image dehazing has been made promising progress with Convolutional Neural Networks (CNNs), the inherent equivariance and locality of convolution still bottleneck dehazing performance. Though Transformer has occupied various computer vision tasks, directly leveraging Transformer for image dehazing is challenging: 1) it tends to result in ambiguous and coarse details that are undesired for image reconstruction; 2) previous position embedding of Transformer is provided in logic or spatial position order that neglects the variational haze densities, which results in the sub-optimal dehazing performance. The key insight of this study is to investigate how to combine CNN and Transformer for image dehazing. To solve the feature inconsistency issue between Transformer and CNN, we propose to modulate CNN features via learning modulation matrices (i.e., coefficient matrix and bias matrix) conditioned on Transformer features instead of simple feature addition or concatenation. The feature modulation naturally inherits the global context modeling capability of Transformer and the local representation capability of CNN. We bring a haze density-related prior into Transformer via a novel transmission-aware 3D position embedding module, which not only provides the relative position but also suggests the haze density of different spatial regions. Extensive experiments demonstrate that our method attains state-of-the-art performance on several image dehazing benchmarks..
Pipeline
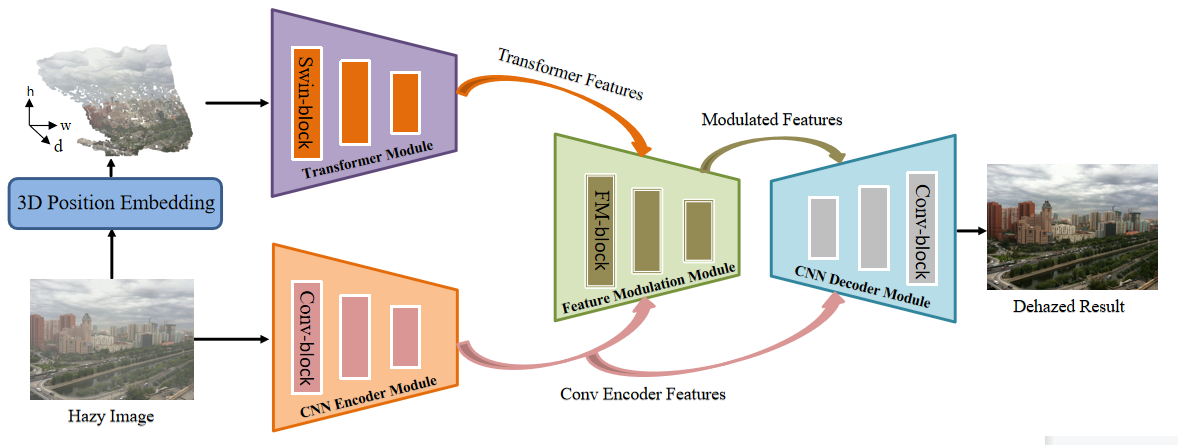
In comparison to pure CNN-based image dehazing networks, our work is the first to introduce the power of Transformer into image dehazing via novel designs.
We propose a novel transmission-aware 3D position embedding to involve haze density-related prior information into Transformer.
Extensive experiments on image dehazing benchmark datasets demonstrate the outstanding performance of our method against state-of-the-art methods.
3D Position Embedding
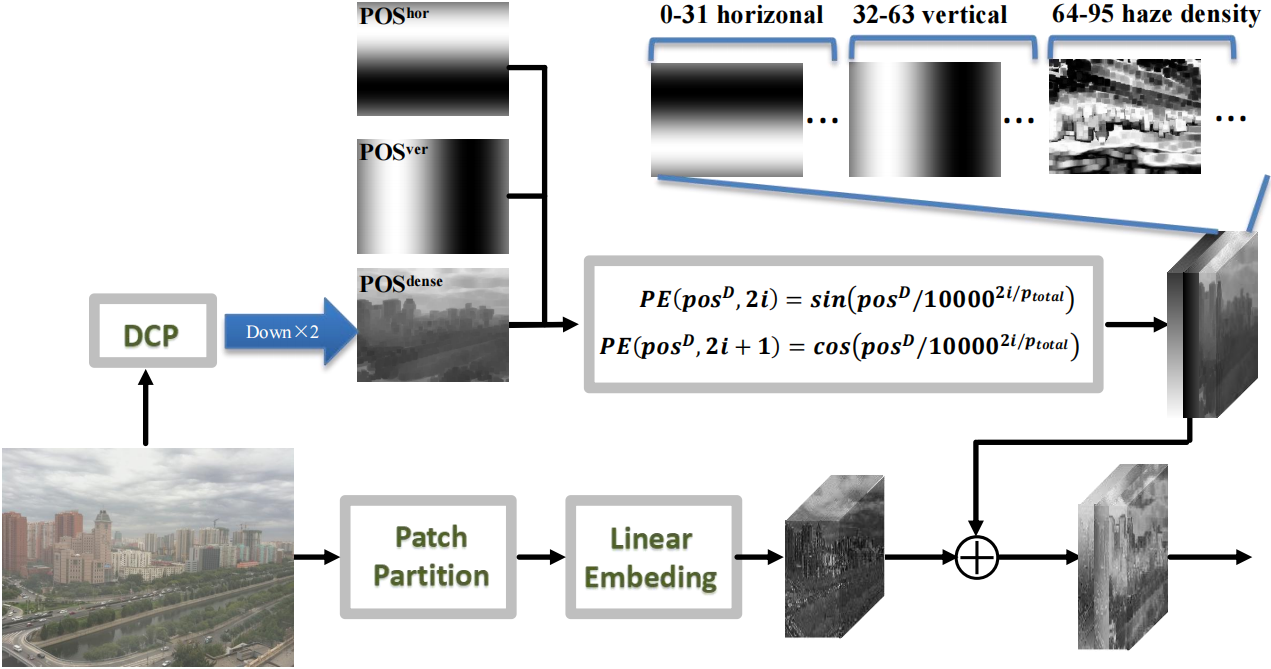
Highlights
Results
1. Visual Comparisons
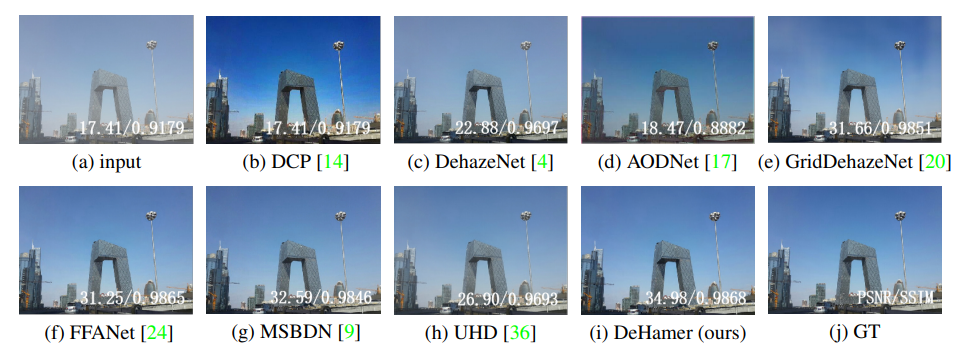
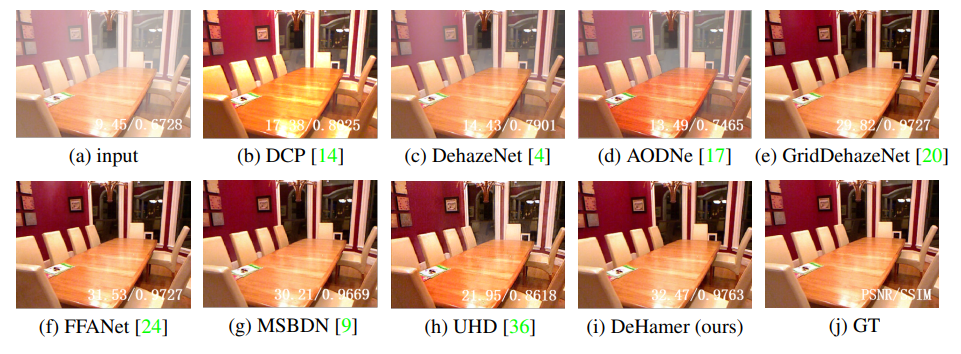
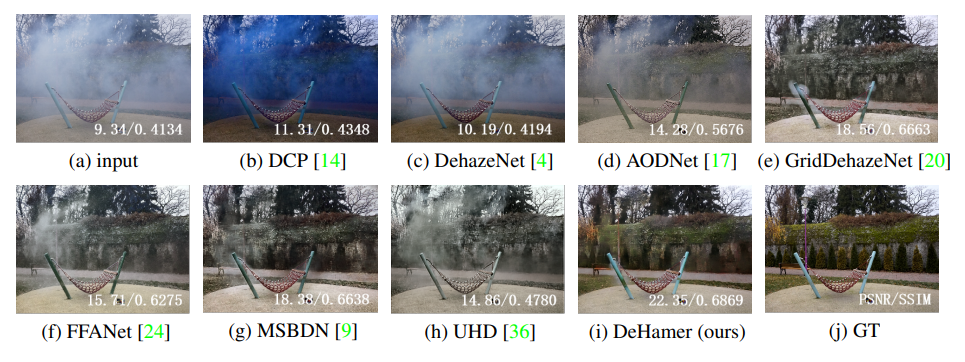
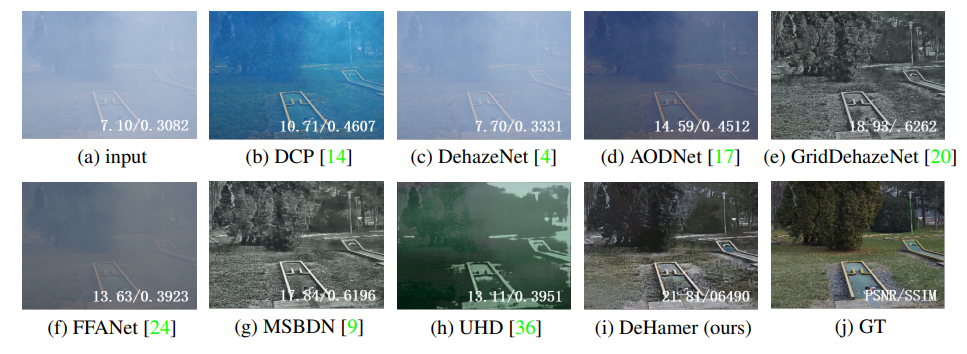
3. Quantitative Results
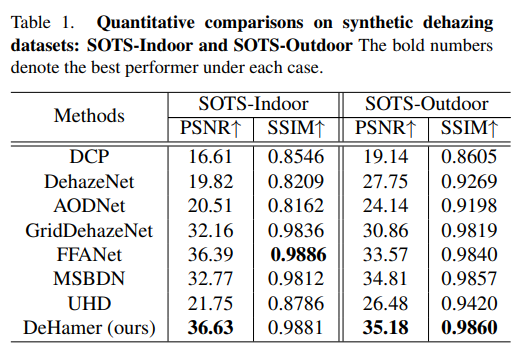
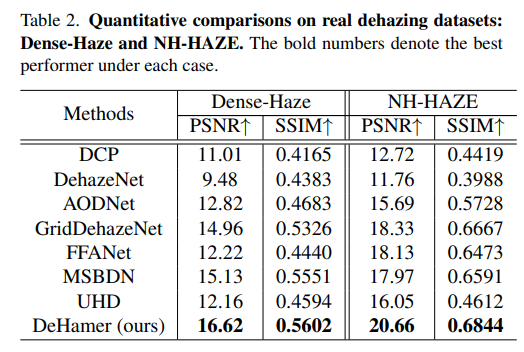
Materials
![]() Paper |
![]() Supplementary Material |
![]() Code and Model |
Citation
@Article{DeHamer2022, author = {Guo, Chunle and Yan, Qixin and Anwar, Saeed and Cong, Runmin and Ren, Wenqi and Li, Chongyi}, title = {Image Dehazing Transformer with Transmission-Aware 3D Position Embeddingn}, journal = {CVPR}, pape={5812-5820}, year = {2022} }
Contact
If you have any questions, please contact Chongyi Li at lichongyi25@gmail.com or Chunle Guo at guochunle@nankai.edu.cn.